By Liz Do
A recent report on the global artificial intelligence (AI) talent pool estimates that there are fewer than 10,000 people worldwide with the competencies necessary to undertake advanced research in AI.
With demand for talent outpacing supply, U of T Engineering continues to create opportunities to prepare engineers to lead and contribute their expertise in this booming field. On Nov. 22, alumni working in data analytics and AI are coming back to Skule™ to network with current graduate students and discuss careers in the industry. Experts on new approaches and emerging applications for AI will share their diverse experiences in areas from blockchain to telecommunications.
Gong was among the first class to graduate from U of T Engineering’s robotics major in Engineering Science, and was also part of aUToronto, the award-winning student team working to turn an electric vehicle fully autonomous as part of the three-year international Autodrive Challenge.
Gong recently spoke with Liz Do to share her career journey so far, and how she applied her U of T Engineering experience into a career in data analytics and AI.
What sparked your interest in autonomous vehicles?
I initially wanted to do a biomedical engineering major, but then the robotics major had just launched at U of T Engineering and I thought, why not try it. Studying the major made me want to dig deeper into robotics and all aspects of programming. This led me to pursue my Professional Experience Year Co-op Program (PEY Co-op) at Zebra Technologies.
Reading and learning on my commute on public transit brought me lots of growth, but its schedule constraints also annoyed me, which made me think more about self-driving cars. I then went on to join aUToronto, and this further developed my interest in autonomous driving and led me onto my current role at General Motors.
What do you do at GM?
I’m working to further develop Super Cruise technology at GM. Super Cruise is an assistive-driving technology, allowing drivers to take their hands off the wheel on mapped, divided highways. It’s semi-autonomous because there is still a camera that monitors drivers’ eyes to keep them engaged – you can’t just go to the back and read a book yet.
A lot of companies’ efforts into creating semi-autonomous or autonomous driving technologies have faced challenges, such as the risk for car accidents due to a driver’s lack of attention. Our technology makes sure that drivers can only enable the function when they are actually on the highway – which is a controlled environment without stop signs or pedestrians.
My particular work is currently on global localization of a vehicle on our high-definition maps to enable more active safety and navigation features in the future.
Right now, this technology is only in one high-end model of Cadillacs but we will definitely extend the coverage in the future. It’s an exciting time to be in the automotive industry.
How has data analytics changed your industry?
I see that a lot of potential ideas have been inspired by the tech industry, and that the automotive industry is keen to innovate and make use of advanced theories. An existing example is the implementation of Waze, which crowd-sources traffic data to help drivers avoid traffic and get home sooner — crowd-sourcing makes heavy use of data analytics knowledge.
The automotive industry is starting to realize that we can use data and AI to improve the experience of our day-to-day travels, and our team is already working towards many future features inspired by it.
Why is it important for graduate students to prepare themselves in the area of data analytics and AI?
There are already many senior engineers who have worked in this industry for decades. When junior engineers, like me, join the automotive industry, we have a lot of experience to gain, but at the same time we can provide insights into cutting-edge algorithms, new technologies, new ways to solve problems. This is definitely the case if you are graduating with the deep knowledge of robotics, or machine learning or AI.
Being able to implement algorithms in the most efficient and robust ways are even more important than ideas themselves, because the products you build are not just for experiments – there will be costs to the company and possibly to society if it fails. Solid implementation skills like coding are not just the means to an end, it’s what turns a dream into reality.
I think it’s also more important than ever for our generation to continue to build their unique perspectives and stay at the cutting edge with life-long learning, because technology has been advancing at a very rapid speed. Our abilities to learn and grow is how we will change the industry, whether that is in automotive or elsewhere.
From blockchain to autonomous driving, alumni share insights on exploding field of AI and data analytics
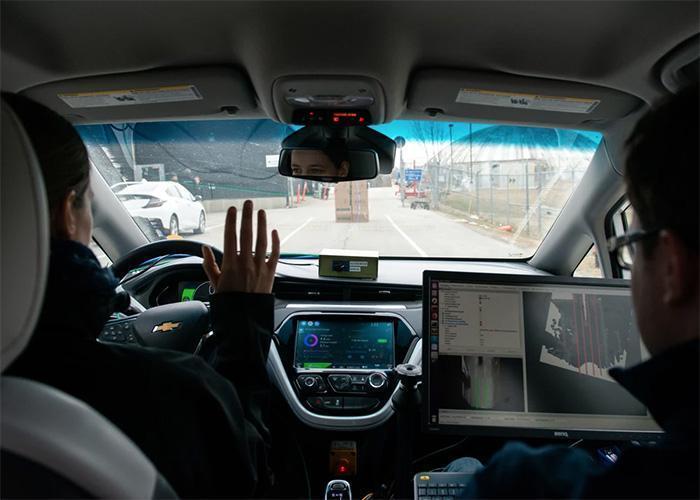